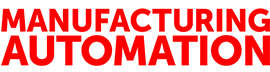
The big picture: Trends in artificial intelligence for manufacturers
November 29, 2022
By Stephanie Holko, NGen

Artificial Intelligence (AI) is touted as a panacea by many. The truth is always more nuanced than that, but AI is a remedy that is a powerful tool if applied correctly. When technology reaches critical mass, there are tools and strategies to support widespread adoption that ease the transition. In this article, I highlight some trends in artificial intelligence technology for manufacturers that are paving the way for widespread adoption and impact in this area. While none of these ideas are theoretically new, they are being applied on a wider scale and at economically attractive price points that make AI accessible in an exciting new context. The solutions in development are across the manufacturing value chain. AI can be seemingly small, embedded in process inputs via sensing technologies. AI can be overarching, providing optimization across multiple operations.
At the input level, AI is now consistently used in sensing applications to mimic human sensing, processing and meaning assignment. These could be vision solutions – telling you what kind of product or defect is present and then deciding what kind of actions or procedures to implement, just like noticing the pizza you put in the oven needs a few extra minutes to come out perfectly cooked. These can be auditory, picking up on those noises that a seasoned operator or tradesperson could pick up when walking around the plant and alerting personnel to an irregularity. The sensing applications can be olfactory, such as deciding a batch of beer is done and needs to be decanted to the next process step. It’s the integration of these sensors into a wider process that is changing how things are made, saving money, reducing waste and minimizing human intervention when not required.
At the plant optimization level, AI augments existing technology. An IoT-based plant advisor system could be even more effective with AI models that can optimize over time for a better process outcome. AI can predict maintenance failures earlier and schedule a diagnostic or repair at a convenient, or non-bottleneck time. It can afford operators the ability to be unceasing eyes and ears (and nose) on the plant and it frees them up for higher-level tasks. AI technology can provide a similar level of diligence without the operator standing over the machine full-time.
Making AI usable is allowing average process specialists take advantage of its power.
AI plant models can be stand-alone. The model optimizes for one outcome, such as uptime or quality or cost. What is emerging is how multiple optimization models can be interlinked and the entire process optimized based on a system of constraints. For example, while you may want perfect quality, that may trigger a cost constraint. It may make business sense to achieve 95 percent quality to minimize the costs of rework. So, 95 percent should be the constraint on the quality model and once that threshold is reached, you can optimize for increased production speed instead. The interplay between AI models at this level is an exciting trend that is just starting to take off.
In the past, one limiting factor of AI adoption was the access to AI development experts who not only know the theory of model development but can code and integrate systems. This bottleneck is prohibitive for many companies for a variety of reasons. Many companies do not have a large enough employee base to justify a full-time resource to support AI development. Luckily, there are several ways that technology is changing that make AI approachable. One way is the development of low-code or no-code solutions that integrate with existing data architectures that allow users with a good understanding of theory to create and tune models for a variety of process and maintenance applications. For example, one solution is an add-on module that connects directly with the plant controls and allows the process specialist to drag and drop data representing good or poor operations into a model. The software trains the model on how to predict issues specific to that plant and recommends actions without having to code anything. This democratization of AI model tuning makes it easy for an average process specialist to take advantage of the power of AI.
AI is a powerful tool that is transforming our manufacturing processes and ways of working. Making AI approachable and usable by plant experts is a fantastic way to meet in the middle of manufacturing expertise and technology with some truly inspiring results on the horizon. Next Generation Manufacturing Canada (NGen) is working to support manufacturers and technology providers in AI innovation. Please connect with us to learn more at info@ngen.ca.
Stephanie Holko is the director of project development at Next Generation Manufacturing Canada (NGen). She loves connecting emerging technologies with existing manufacturing problems and believes the future of manufacturing is in the adoption of new ways of working. Stephanie is a licensed Professional Engineer in Ontario.
This article was first published in the September/October 2022 issue of Manufacturing AUTOMATION.
Advertisement
- LoRa Alliance certification program now includes SCHC over LoRaWAN testing
- Omron names PPD Automation as latest Certified Systems Integrator partner